01
Τo overcome the limited amount of data typically available in radiomics, INFORM investigates Monte Carlo (MC) simulations combined with generative adversarial networks (GAN) for producing large amounts of highly realistic simulated images to facilitate training DNNs.
02
We tackle the interpretability of DNN-based feature engineering and latent variable modeling with innovative developments of saliency maps and related approaches for relevance scores.
03
INFORM proposes to build explainable AI models that incorporate both conventional radiomics and DNN-based features.
04
Preliminary evaluation will be carried out with the help of clinical collaborators on predicting outcome of patients with lung, cervical and rectal cancer. Leverage the robustness and trustworthiness of expert-derived features.
Overall, INFORM’s methodological research and clinical application will advance interpretability of feature engineering, generative models, and DNN classifiers with applications in radiomics and broad medical imaging.
Our team of Big Thinkers
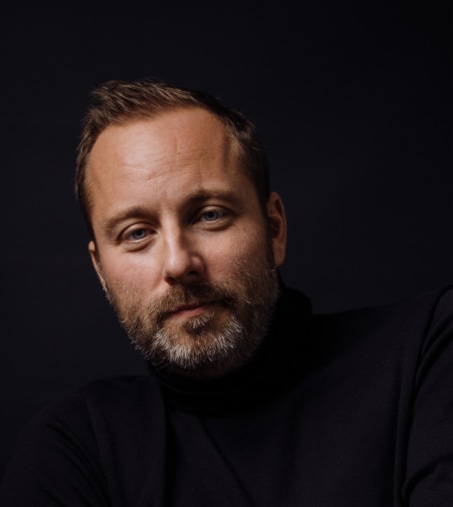
Rudy Horne
Founder, Brand Strategist
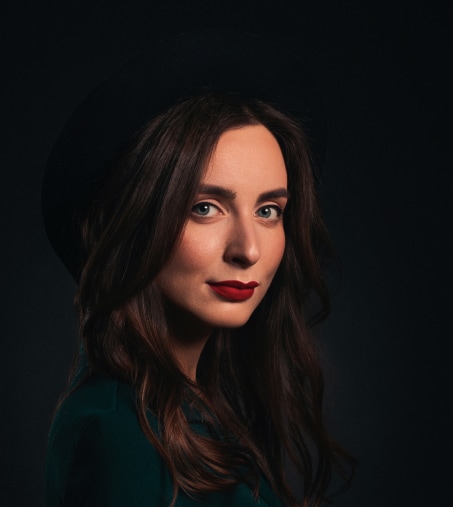
Sabrina Philip
Creative Director
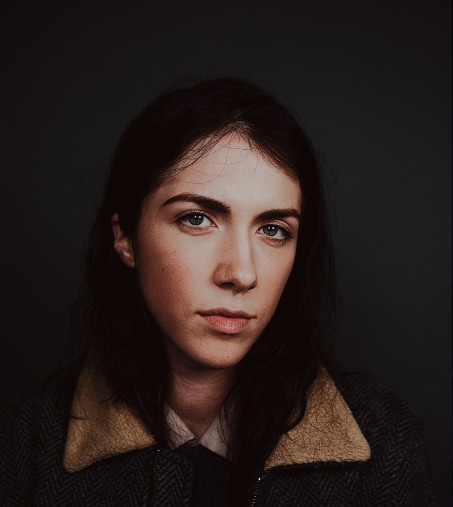
Teegan Squires
Web Designer
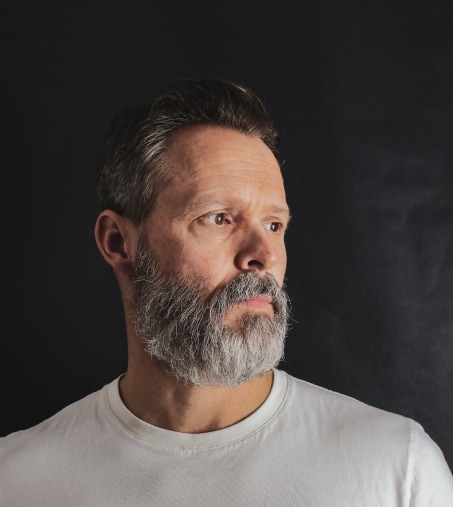
Cruz Lowry
Digital Marketing Manager
This project is supported by the CHIST-ERA grant [CHIST-ERA-19-XAI-007] with project acronym INFORM, by:
General Secretariat for Research and Innovation (GSRI) of Greece [T12ΕΡΑ5-00053],
National Science Centre (NCN) of Poland [2020/02/Y/ST6/00071] and,
Agence Nationale de la Recherche (ANR) of France [ANR-21-CHR4-0006].
supported by
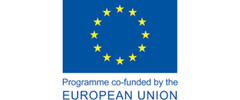
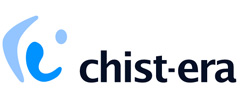
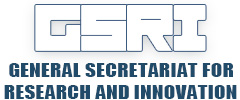
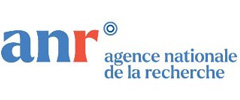
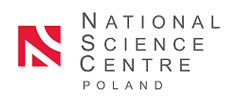
Let’s get creative
Lorem ipsum dolor sit amet, consectetur adipiscing elit. Sed auctor turpis eu arcu sagittis, id sagittis justo suscipit. Aliquam erat volutpat. Integer finibus sem
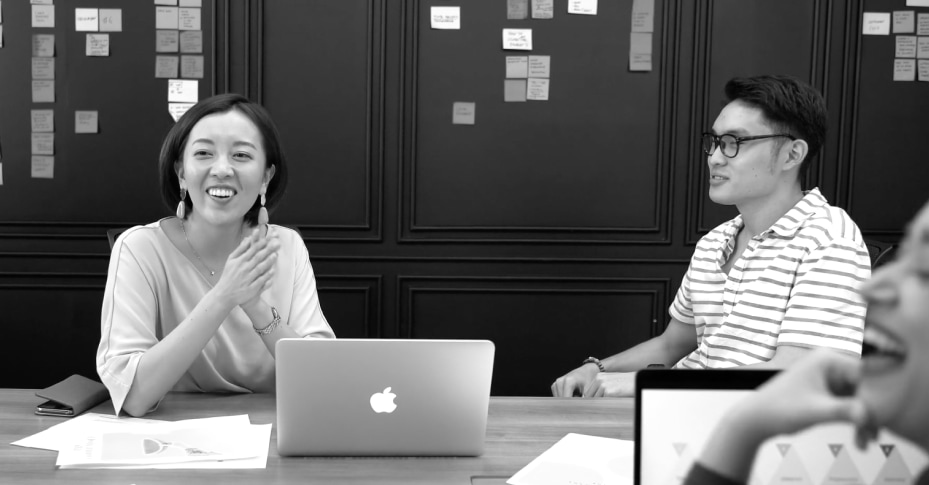
© 2025 All Rights Reserved.