background
Radiomics is defined as the high-throughput extraction of “engineered/handcrafted” features from medical images. It has the potential to provide a quantitative signature of tumors’ characteristics that cannot be appreciated visually and has shown promising results in identifying tumor subtypes and in predicting outcome by relying on ML methods to exploit radiomic features in combination with clinical or other variables to build predictive models. The use of DL and specifically convolutional neural networks (CNNs) in computer vision have led to state-of-the-art results in filtering, segmentation, classification, and synthesis (image-to-image translation) including for medical images. However, the attempts for predictive modeling in radiomics were met with limited success given the limited amount of available data for training compared to other applications. Nonetheless, the current research trend is to rely on DL as it allows a higher level of automation compared to the traditional workflow and may therefore facilitate its clinical translation.
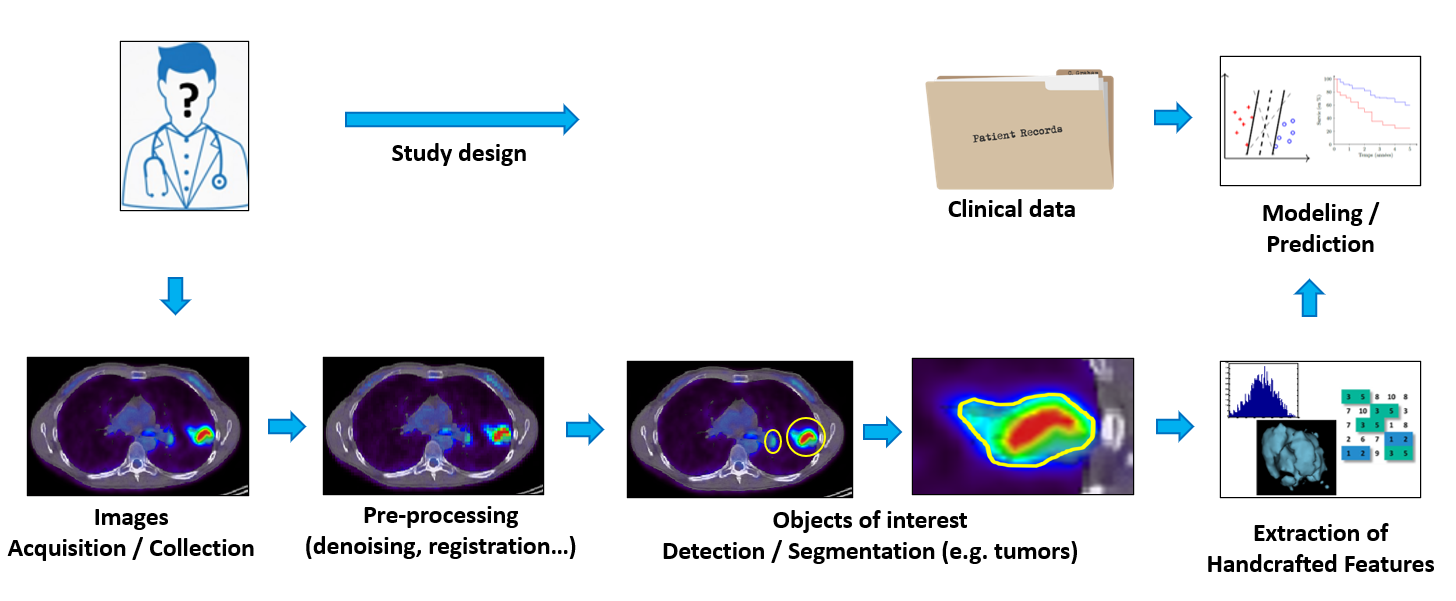
It is challenging for end-users to interpret multiparametric radiomic models and grasp the mathematical and algorithmic implications of radiomics. The issue of acceptability will therefore become even more critical with DNN-based models, with millions of parameters. Within this context, we first aim to achieve an efficient training of DNNs with limited available data for predicting outcomes. We then aim at making the prediction made by the model both clinically relevant and explainable, such that it is trusted and understood by end-users (i.e. clinicians). The INFORM project aims to develop paths away from unexplainable black box algorithms towards explainable grey/white box models for radiomics applications.
supported by
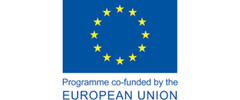
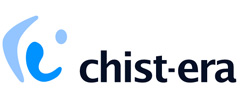
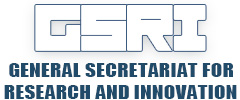
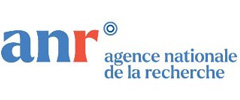
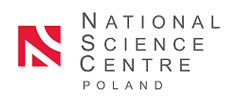