Work Packages
The project is structured in 5 Work Packages (WPs). WP1 deals with the overall management and dissemination of all the activities. WP2 & WP3 are designed in order to feed WP4 & WP5, that are the main core of the project, as they include all the new developments and training processes in terms of explainable AI.
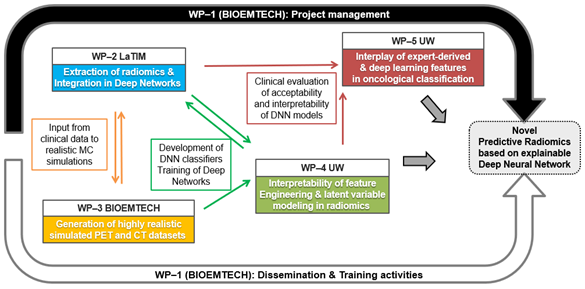
Main objective of this WP is to ensure the smooth implementation of the project and the good collaboration between the partners. In addition, there are all the appropriate actions for the increase of the impact of the project outcomes, as well as to extensively disseminate the results and explore the exploitation potentiality.
This WP supervises the extraction of radiomic knowledge from the datasets and how it can be integrated within deep networks to facilitate and constrain their training.
The aim is to combine GAN-type architectures with MCS, in order to produce large datasets of realistic, synthetic medical CT and PET images with tunable and quantitative preserved radiomic features. The generated data will be subsequently used for training of deep networks and transfer learning
Modern machine learning methods, such as (DNNs), have enabled discovering the latent space of cancer and automatic feature engineering from medical images. DNNs for object classification and detection have been successful largely due to how the features are created and used from input pixel values. Furthermore, we have observed that deep latent variable models such as variational auto-encoders (VAE) are able to automatically generate meaningful features that can be directly fed into classification algorithms. However, their translational values and clinical adaptations are limited due to a lack of interpretability of features and latent variables derived from deep learning. The main objective of this WP is to investigate and develop novel methods to aid our understanding of DNNs in radiomics
We aim at understanding how DNN-based features can be partially explained by well-known radiomic and/or expert-derived features, and vice-versa. By developing informative and transparent ways to incorporate these features, the proposed methods will take advantage of expert knowledge and automated feature engineering based on deep learning. This will be increasingly helpful to clinicians with broad acceptance of existing computing systems and manual features.
WP 1
Project management / Dissemination and Training activities (BIOEMTECH)
Main objective of this WP is to ensure the smooth implementation of the project and the good collaboration between the partners. In addition, there are all the appropriate actions for the increase of the impact of the project outcomes, as well as to extensively disseminate the results and explore the exploitation potentiality.
WP 2
Extraction of radiomics and expert knowledge for integration into deep networks based modeling (LATIM)
This WP supervises the extraction of radiomic knowledge from the datasets and how it can be integrated within deep networks to facilitate and constrain their training.
WP 3
Generation of highly realistic simulated PET and CT datasets (BIOEMTECH)
The aim is to combine GAN-type architectures with MCS, in order to produce large datasets of realistic, synthetic medical CT and PET images with tunable and quantitative preserved radiomic features. The generated data will be subsequently used for training of deep networks and transfer learning
WP 4
Interpretability of feature engineering and latent variable modeling in radiomics (UW)
Modern machine learning methods, such as (DNNs), have enabled discovering the latent space of cancer and automatic feature engineering from medical images. DNNs for object classification and detection have been successful largely due to how the features are created and used from input pixel values. Furthermore, we have observed that deep latent variable models such as variational auto-encoders (VAE) are able to automatically generate meaningful features that can be directly fed into classification algorithms. However, their translational values and clinical adaptations are limited due to a lack of interpretability of features and latent variables derived from deep learning. The main objective of this WP is to investigate and develop novel methods to aid our understanding of DNNs in radiomics
WP 5
Interplay of expert-derived and DL features (UW)
We aim at understanding how DNN-based features can be partially explained by well-known radiomic and/or expert-derived features, and vice-versa. By developing informative and transparent ways to incorporate these features, the proposed methods will take advantage of expert knowledge and automated feature engineering based on deep learning. This will be increasingly helpful to clinicians with broad acceptance of existing computing systems and manual features.
supported by
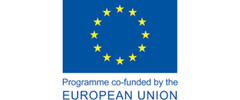
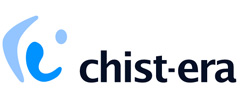
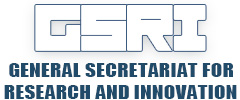
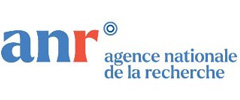
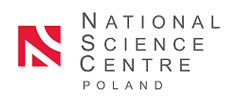